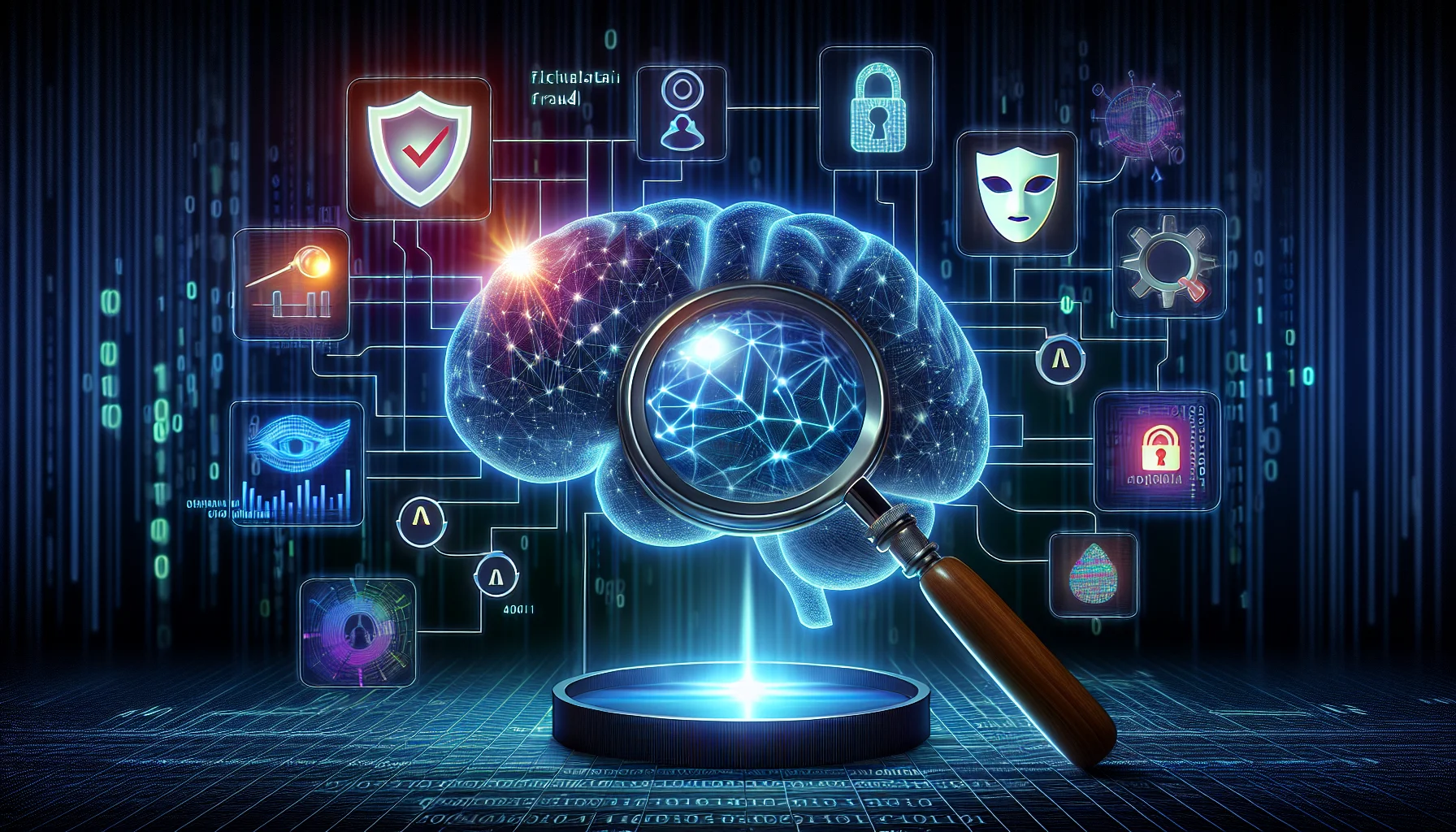
はじめに
今日のデジタル時代において、金融取引は迅速かつ頻繁に行われている。取引量とスピードが増すにつれ、金融詐欺の可能性も高まっています。サイバー犯罪者は、常に悪用できる脆弱性を探しているため、詐欺師を出し抜くことができる最新かつ効果的な詐欺防止ソリューションの必要性が高まっています。そこで AI不正防止 最先端のテクノロジーを駆使して取引データを分析し、不正行為をリアルタイムで防止する。
AI不正防止システムを理解する
AI不正防止システムの定義と役割
AI不正防止 システムは、機械学習や予測分析などの人工知能(AI)技術を採用し、不正取引の検出、防止、管理を行う高度な検証ツールである。これらのシステムは、大量の取引データをふるい分け、不正行為を示す可能性のある異常な行動を特定する。
現代の不正防止におけるAIの必要性
現代の金融システムは複雑であり、サイバー攻撃も蔓延しているため、不正行為を迅速かつ正確に検知できる堅牢でプロアクティブなソリューションの利用が必要となっている。 AI不正防止 システムは、変化するトレンドや脅威に適応できるタイムリーで正確な不正防止を提供し、この増大する課題に対する強力な回答を提供します。
不正検知と防止にAIを採用する主なメリット
AI不正防止 システムは、従来の不正検知方法と比較して多くの利点を提供します。検知時間の短縮、高い精度、膨大なデータをリアルタイムで分析できる能力、新たな不正の脅威への適応性などである。さらに、AIシステムは人間の作業負担を軽減し、戦略的な業務に集中できるようにする。
不正防止における機械学習の役割
機械学習がAIシステムに不正行為を検知させる仕組み
AIの重要な構成要素である機械学習は、過去のデータから学習し、時間の経過とともに検知を改善する能力を不正防止システムに与えます。システムは、不正行為を示す可能性のある取引データのパターンや異常を特定する能力を高め、不正検知の精度と効率を向上させる。
自己学習アルゴリズムが不正検出に与える影響
機械学習モデルの自己学習アルゴリズムは、以下を可能にする。 AI不正防止 システムは常に進化し、金融詐欺の新しいトレンドやテクニックに適応している。これにより、詐欺師がシステムに侵入する新たな方法を編み出しても、詐欺を予測・防止する能力が大幅に向上する。
効果的な不正検知のための機械学習と人間の監視の融合
機械学習が大きく貢献する一方で AI不正防止 システムでは、人間による監視と裁量の重要性を軽視することはできない。これらのシステムは、人間の専門家と連携し、彼らの知性を活用して、情報に基づいた決定を下し、必要に応じて是正措置を取るように設計されている。
リアルタイム・モニタリングと不正防止
不正防止におけるリアルタイム・モニタリングの重要性
リアルタイム・モニタリングは AI不正防止 システムにより、疑わしい行為を即座に検知し、防止することができる。このような迅速な対応は、不正取引による潜在的な損害を最小限に抑えるために不可欠である。
リスク評価におけるAIの統合
リスク評価におけるAIは不可欠なものとなった。企業がリスクを評価し、軽減する方法に根本的な変化をもたらす。
AIがリスク評価にどのような革命をもたらすか
人工知能は、複雑なパターンを理解し、将来のリスクを予測するために、高度なアルゴリズムと機械学習モデルを活用します。膨大な量のデータを迅速に処理し、潜在的な脅威への対策に役立つ優れた洞察力を明らかにします。 AI不正防止 システムは今日、この革命をもたらす重要な役割を果たしている。
リスク評価における予測分析の役割
AIによる予測分析は、過去と現在のデータを効率的に分析し、将来のリスクを予測することができる。このAIを活用した先見性は、不正取引の予測だけでなく、戦略的な予防策の策定にも極めて重要である。
リスク評価とAIの組み合わせで最適な不正防止を実現
AIをリスク評価に統合することで、不正防止が強化される。リスクモデルを精緻化し、精度と拡張性を同時に向上させることで、強固なリスクモデルを構築することができます。 AI不正防止 システムである。
AI不正防止ツールとその応用
AIツールは、高度な不正検知・防止ソリューションを提供することで、さまざまな分野に変革をもたらしつつある。
市場におけるAI不正防止ツールの主な種類
AI不正防止 を搭載したツールもある。 機械学習 アルゴリズム、ニューラルネットワーク、自然言語処理。これらはパターンを分析・学習し、不正行為を予測・防止するようインテリジェントに設計されている。
さまざまなセクターが不正検知にAIをどのように活用しているか
銀行や金融機関は取引データの異常を検出するためにAIを使用し、保険業界は保険金や保険料の不正検出にAIを活用し、eコマースは購入詐欺や偽レビューに対抗するためにAIを採用している。
AI不正防止ツールの将来展望
という需要がある。 AI不正防止 ツールは、巧妙な脅威の蔓延に伴い、今後も成長を続けるだろう。予測分析とディープラーニングの発展により、不正の検出と防止はさらに微調整されるでしょう。
AI不正防止システム導入の課題と解決策
こうした利点がある一方で、AIを活用した不正検知システムには課題もある。
不正検知のためのAI導入に共通するハードル
データの質やプライバシーの問題、熟練した専門家の不足、多額の投資の必要性などが、不正検知のためのAI導入のハードルとなっている。
プライバシーとデータセキュリティの懸念への対応
堅牢なデータ管理とセキュリティ対策は、個人情報保護法の厳格な遵守とともに、プライバシーとデータセキュリティに関する懸念に対処するのに役立つ。また、AIの運用において透明性を重視することで、利用者の信頼を築くことができる。
継続的な学習とシステムの適応性によって課題を克服する
AIシステムは適応性が高く、新たな不正事例から学習できる必要がある。このようなシステムの継続的なアップグレードと熟練した労働力への投資により、導入のほとんどの障壁を克服することができる。